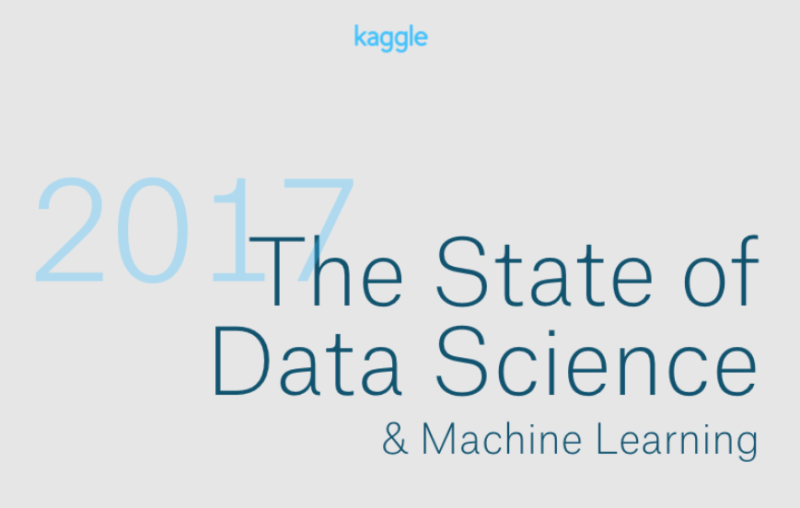
Kaggle recently conducted a survey of 16,000+ data scientists called ‘The State of Data Science & Machine Learning’. In this post we discuss some of the key takeaways that we pulled out of their survey for businesses thinking about leveraging machine learning and data-science technology.
Here are the biggest take-aways that Vidora took from the survey:
- The two largest barriers to the adoption of data science are: (1) Dirty data, and (2) Lack of available data science talent
- The Machine Learning models used by data-scientists vary dramatically across different industries and problem types. There is no unanimous model that always works, meaning ML engineers and data scientists will need to try multiple models for every machine learning problem they tackle
The rest of this post describes the data science challenges in more details and shows how Automated Machine Learning, or AutoML for short, helps businesses of all sizes and industries solve some of these problems.
What Is Kaggle?
Kaggle is the world’s largest community of data scientists, and allows its members to collaborate, learn, and share their work among their peers. The platform also allows companies, researchers, government and other organizations to post their modeling problems and have data professionals and researchers compete to produce the best solutions. Kaggle offers data professionals and researchers the opportunity to test their skills, try their techniques on interesting datasets and enhance their professional reputations.
What Barriers do Businesses Typically have to Adopting Machine Learning?
According to the survey, the largest problem data scientists face is “Dirty Data”. Data scientists spend about 90% of their time cleaning dirty data and creating features from that dirty data. These features then help to train models (the first two steps below in “Feature Cleaning” and “Feature Engineering”) and only ~10% of their time on Model Selection.
Machine Learning algorithms require a few steps. First, you need to clean and normalize data. Secondly, you need to create more complex features simpler base features. Finally, select the best model to learn based on that data. When an algorithm automates these steps of data preparation and model selection it is known as Automated Machine Learning (AutoML).
The next largest barrier according to the survey is the lack of data science talent. This is a problem because there are too many Machine Learning problems to tackle, and not enough engineers available to tackle them. But Cortex’s AutoML framework allows organizations to automate the training of some machine learning models thereby expanding the scope of what the existing data science team can engage on.
Barriers to Entry: Kaggle’s survey results showing the most common reasons businesses have trouble adopting machine learning technology. Note that the top two barriers are (1) Dirty Data and (2) Lack of Data Science Talent. Vidora Cortex addresses both.
What Machine Learning Models are Most Common?
The survey also analyzed the most popular machine learning models among data scientists. Here is our analysis of the results:
- Different deployments need different models. As we know, in general, it’s hard to predict which model will work best for a particular machine learning problem. Therefore with AutoML, we try multiple models for each problem. We do this in order to determine which will work best for that particular ML problem.
- Regression is one of the more traditional machine learning models. This is also the most common model. Therefore, this shows that many ML engineers are not engaging with some of the newer machine learning techniques.
Solving ML Problems: Kaggle’s survey results showing which ML models are most commonly used to solve business problems. In general it is good to try multiple different models. This is because you will have trouble knowing a priori which model will work best.
Vidora Cortex for Automated Machine Learning (AutoML)
The Kaggle survey shines a light on many of the challenges facing businesses today in deploying machine learning technology. Vidora Cortex, and it’s associated AutoML engine, helps quickly realize the benefits of machine learning technology. You can learn more about Cortex and Automated Machine Learning by contacting us at info@vidora.com.