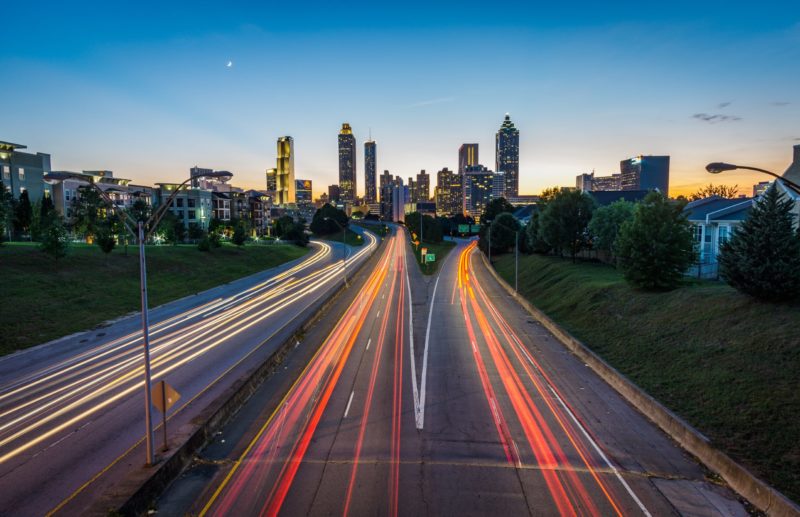
How is your business using machine learning?
When working with customers we often see two extremes in how a business thinks about machine learning – Analysis and Automation. These two ways businesses use machine learning typically require very different technology stacks and cater to different types of business users.
Let’s spend a few minutes to dive into the differences!
Machine Learning for Analysis
When machine learning is used for analysis, it’s typically to deeply understand a business problem. Usually, the cadence of receiving new data is slower than in the automation use case. In addition, the cadence of making a decision based on that data is slower. The goal here is to learn machine learning to better understand the outcome of a future decision.
Technology Stack
The tools needed in the analysis case involve deeply understanding the data and visualizing various components of the data. This includes not only the output of the machine learning model, but also how the model came to a particular decision. These types of use cases often require a deep understanding of the features used by the model and their relative strengths.
Machine Learning for Automation
In automation cases, machine learning is used to automate a task within a business and, in the process, leverage the inherent strengths of machine learning like continual learning based on changing market conditions. Typically, these machine learning systems are constantly presented with new data and asked to make decisions on an ongoing basis. In this case, the business goal is to ensure the optimal business decision is being made today and in the future by learning from the existing data.
Technology Stack
Automation use cases usually require different types of machine learning stacks. In particular, real-time machine learning is an important consideration (Vidora product manager Michael Firn has a good write-up here on real-time machine learning) – the system should not only be able to make decisions quickly but also incorporate new data quickly. In addition, monitoring and the field of ML Operations (MLOps) becomes important when a model is live in a production setting automating a business task.
Note that some analysis of the models is also needed when implementing machine learning automation. Specifically, understanding which features are important can help a business know whether the model is learning the right business use case.
Summary – Analysis vs. Automation
What about Vidora?
At Vidora, we focus on machine learning for automation. Our Cortex platform focuses on enabling ongoing business automation and the tools needed to ensure that the automation performs well over time. That being said, Cortex does provide some tools for visualizing how models are making decisions and exporting data to dive deeper into models. However, the core focus is for enabling businesses to deploy high quality automating machine learning technology.
Examples – Analysis vs. Automation
Wrapping it Up
As businesses become more familiar with integrating machine learning, the nuances of how machine learning is used become more apparent. When engaging in your next machine learning project it may be useful to consider whether you are interested in analyzing a problem more deeply or automating some aspect of your business. The answer to this question will help determine what tools and techniques you should be using. Please contact us at info@vidora.com for any questions!